近日,广州海洋实验室唐丹玲U团队利用机器学习预测热带气旋风泵引起的海表温度响应研究取得进展,相关成果以“Predicting tropical cyclone-induced sea surface temperature responses using machine learning”为题发表在SCI 1区期刊Geophysical Research Letters(影响因子:5.2)上,并作为亮点新闻被Phys.org与CityLife等多家国际科研资讯媒体报道。论文第一作者为广州海洋实验室-香港科技大学联培博士研究生崔红星,通讯作者为广州海洋实验室教授、广东省海洋生态环境遥感中心唐丹玲博士。合作单位包括美国北卡罗来纳大学教堂山分校、加拿大达尔豪斯大学与英国肯特大学等。
研究采用随机森林方法及一种基于机器学习的高效方法构建了一个模型。该模型的目标是预测西北太平洋热带气旋(TC)引起的海面温度(SST)冷却的空间结构和时间演变,即风泵过程。在模型的构建过程中,利用了与热带气旋特征和热带气旋前海洋状态相关的12个变量作为预测因子。研究结果显示,这一模型能够很好地预测不同强度组的热带气旋所产生的冷尾流的时空演变,并成功捕捉到观测的SST响应在不同热带气旋之间的变异。为了进一步评估12个预测因子在确定最大降温幅度方面的相对重要性,采用相同的机器学习方法建立了另一个模型。通过对预测因子特征得分的计算,结果表明在考虑降温区域大小时,热带气旋尺度、强度、平移速度以及热带气旋来临前混合层深度和海表温度在确定最大降温幅度方面占主导地位。总的来说,本研究证明机器学习在预测热带气旋引起的SST响应以及评估热带气旋引起的SST冷却过程中的主导因子具有很大潜力。
唐丹玲团队长期研究海洋风泵的生态环境效应,该研究方法和结果对于预测热带气旋风泵效应所引起的海洋初级生产的变化具有重要意义,将有助于防范极端事件(如风暴潮)对人和财产的影响。
该研究由广东省特支计划U团队项目(2019BT02H594)、广州海洋实验室人才科研启动项目(GML2021GD0810)和2022年度南沙区重点领域科技项目(2022ZD003)等资助。
媒体报道:
https://phys.org/news/2023-09-ai-sea-surface-temperature-cooling.html
https://citylife.capetown/science/ai-predicts-sea-surface-temperature-cooling-during-tropical-cyclones/392213/
论文全文:Cui, H., Tang, D., Mei, W., Liu, H., Sui, Y., & Gu, X. (2023). Predicting tropical cyclone-induced sea surface temperature responses using machine learning. Geophysical Research Letters, 50, e2023GL104171. https://doi.org/10.1029/2023GL104171
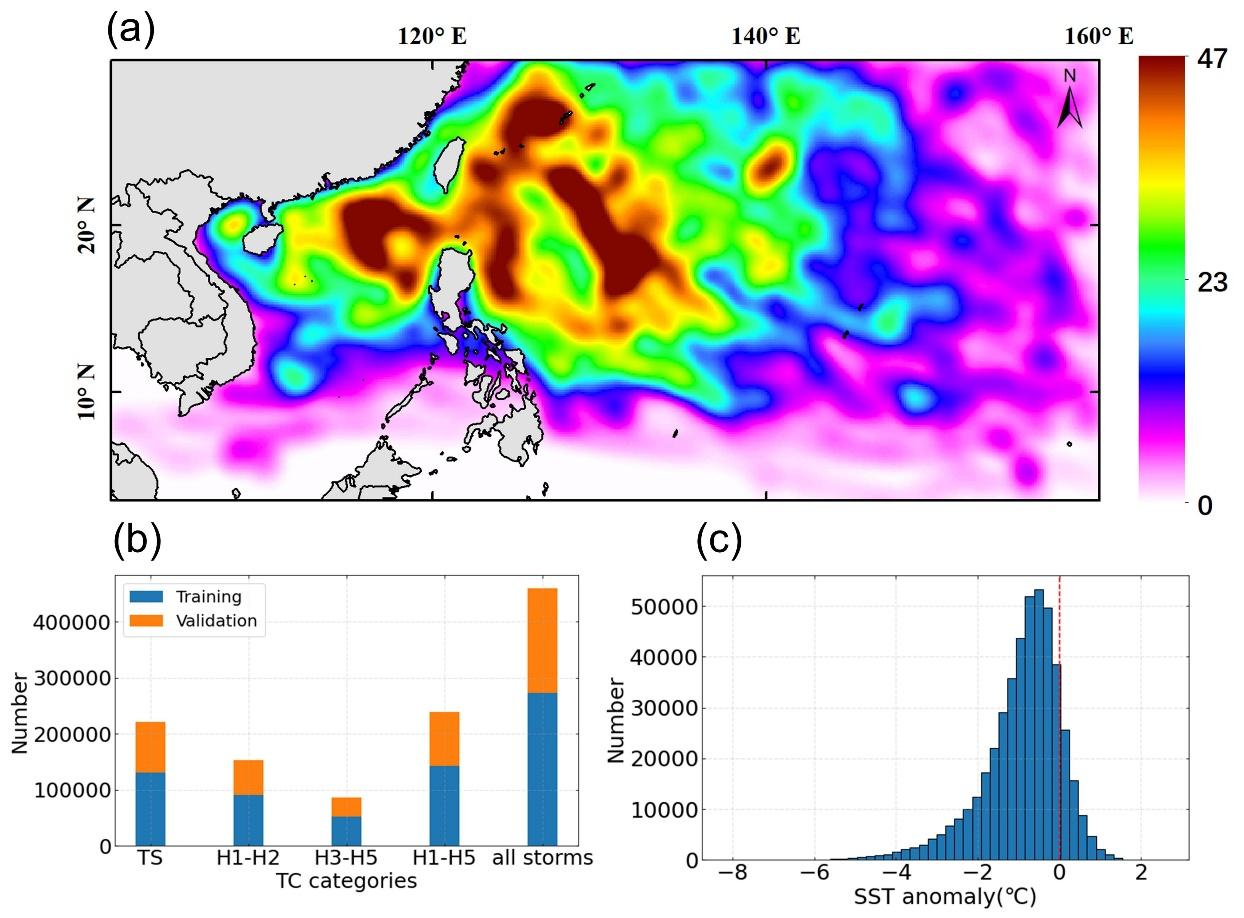
Figure 1. Study area and distribution of data points in the training and validation sets during January 1998–December 2018. (a) TC occurrence density, calculated as the number of TC occurrence during the entire study period within individual 0.1°×0.1° grids. (b) Histogram of the number of data points used in the training stage as a function of TC intensity. (c) Histogram of TC-induced SST anomalies on day 1 after TC passage in the training stage. Abbreviations: TS, tropical storm; H1–H2, category-1–2 hurricane; H3–H5, category-3–5 hurricane; and H1–H5, category-1–5 hurricane.
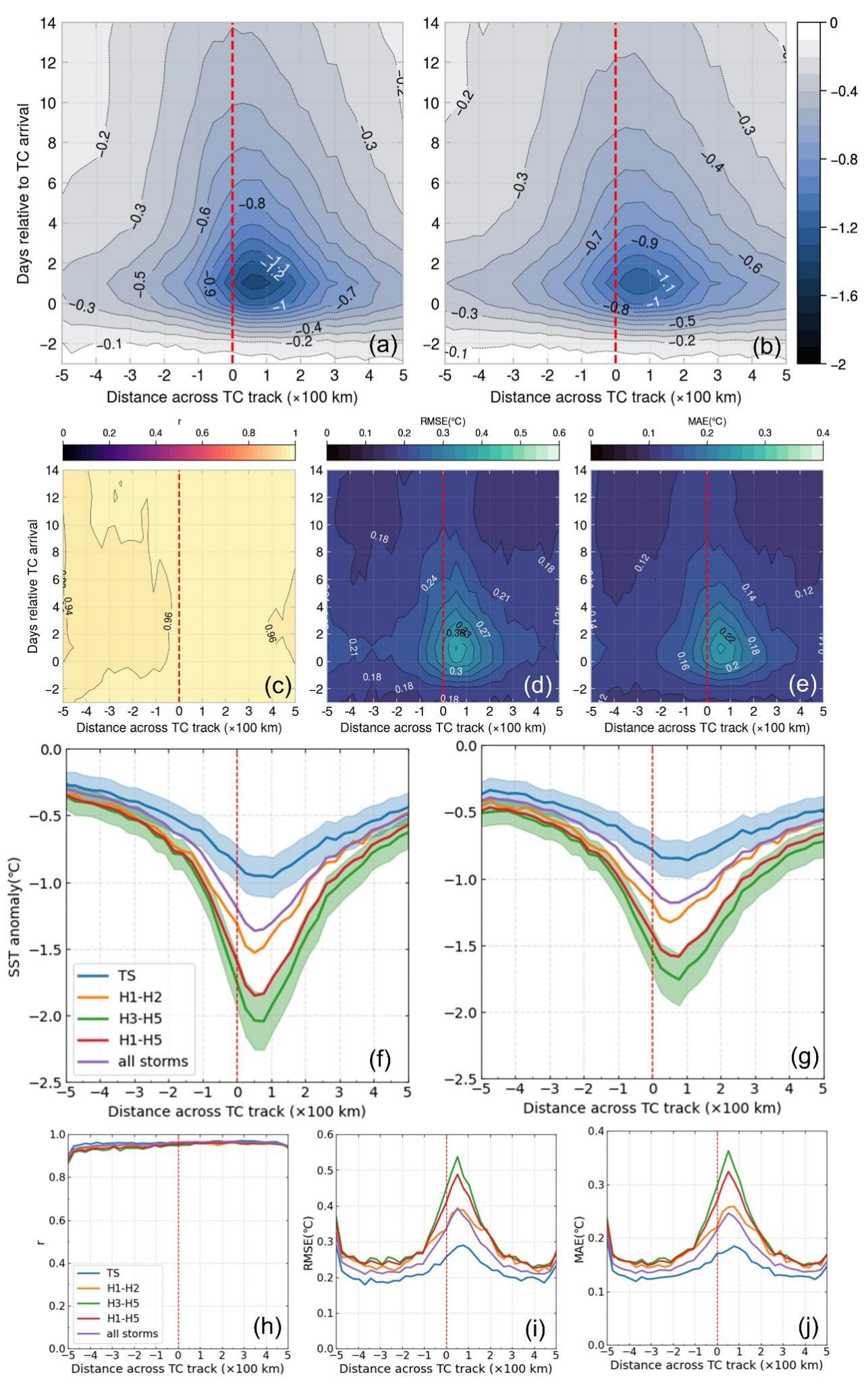
Figure 2. (a) Spatial structure and temporal evolution of the TC-induced SST anomalies (℃) in the observations from the testing set. (b) As in (a), but for the predictions. (c)-(e) Evaluation of the model performance for (b), i.e., (c) correlation coefficient r, (d) RMSE (℃), and (e) MAE (℃). (f) TC-induced SST anomalies in the observations as a function of the distance across TC track on the day right after the cyclone passage when the SST anomaly near the storm center peaks. Only the standard errors for TS and H3–H5 are shown (light blue and light green shading, respectively), and are calculated as the standard deviation divided by the square root of the number of observations used in the testing stage (i.e., standard error of the mean); the size of the standard errors for the other three intensity groups is in between these two. (g) As in (f), but for the predictions. (h)-(j) Evaluation of the model performance for (g), i.e., (h) correlation coefficient r, (i) RMSE (℃) and (j) MAE (℃), for different TC intensity groups.
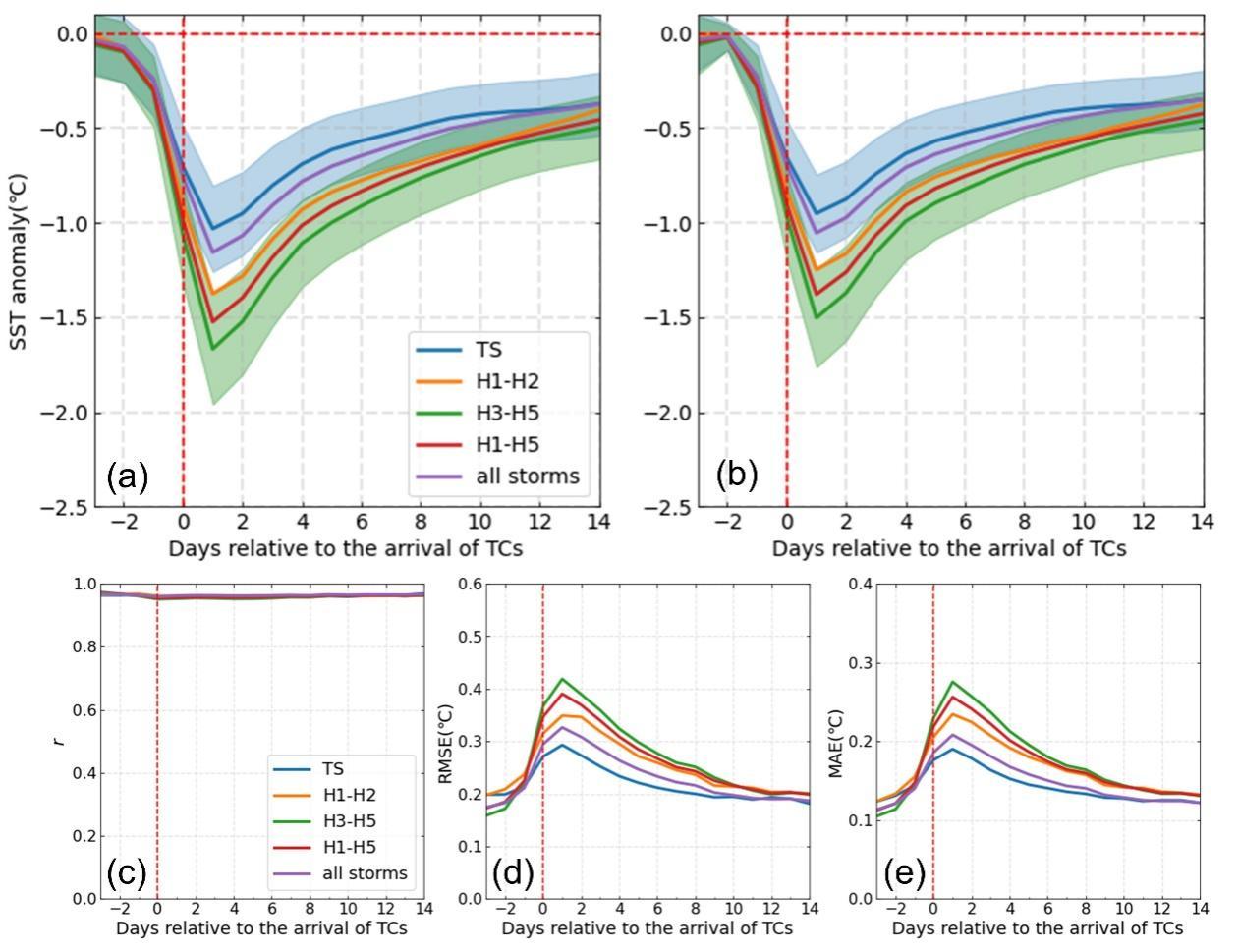
Figure 3. (a) Temporal evolution of the composite SST anomaly averaged within a TC-centered 3°×1° box in association with the passage of TCs of different intensity groups in the observations from the testing set. The standard errors for TS and H3–H5 are calculated and shown as in Figure 2(f). (b) As in (a), but for the predictions. (c)-(e) Evaluation of the model performance for (b), i.e., (c) correlation coefficient r, (d) RMSE (℃) and (e) MAE (℃), for different TC intensity groups.
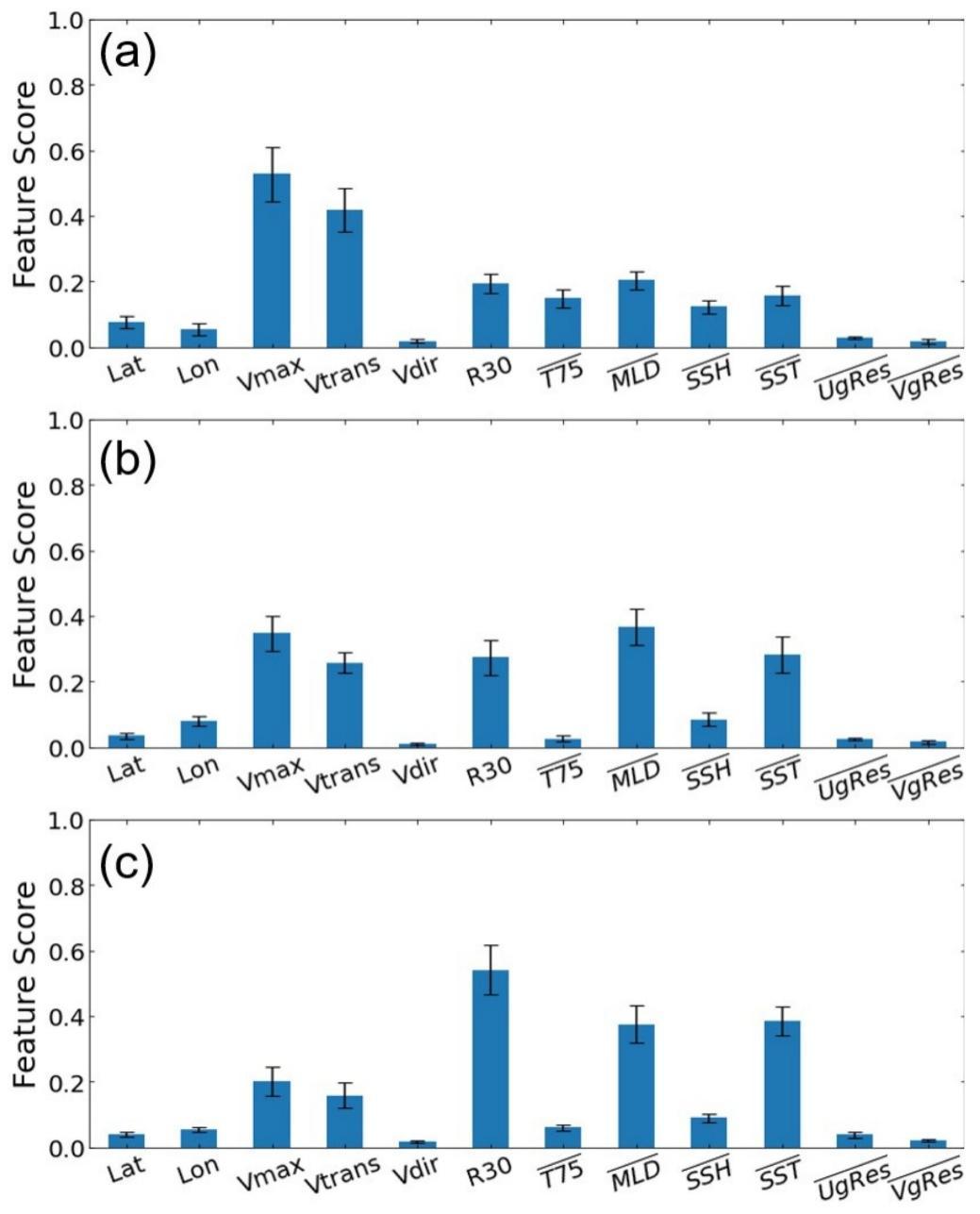
Figure 4. (a) The feature scores of the 12 predictors used in the model constructed to predict the maximum SST cooling induced by TCs averaged within a TC-centered 1°×1° box. (b),(c) As in (a), but for the model constructed to predict the maximum SST cooling induced by TCs averaged within TC-centered 3°×3° and 5°×5° boxes, respectively. Vertical lines show the standard deviations of the feature scores.
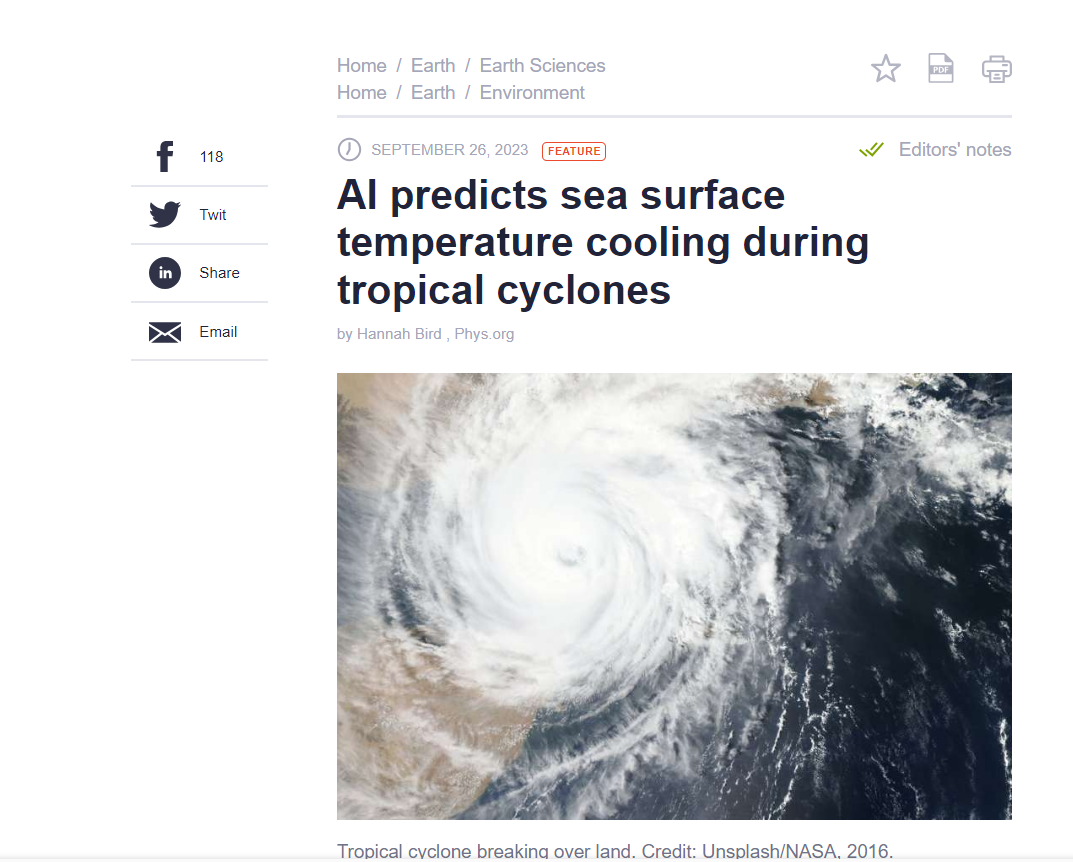
Phys.org报道(亮点新闻)